Research
ArcticWATCH
Early Warning of Future Rapid Arctic Sea Ice Loss
Contributors
François Massonnet Patricia DeRepentigny Alison Delhasse Annelies Sticker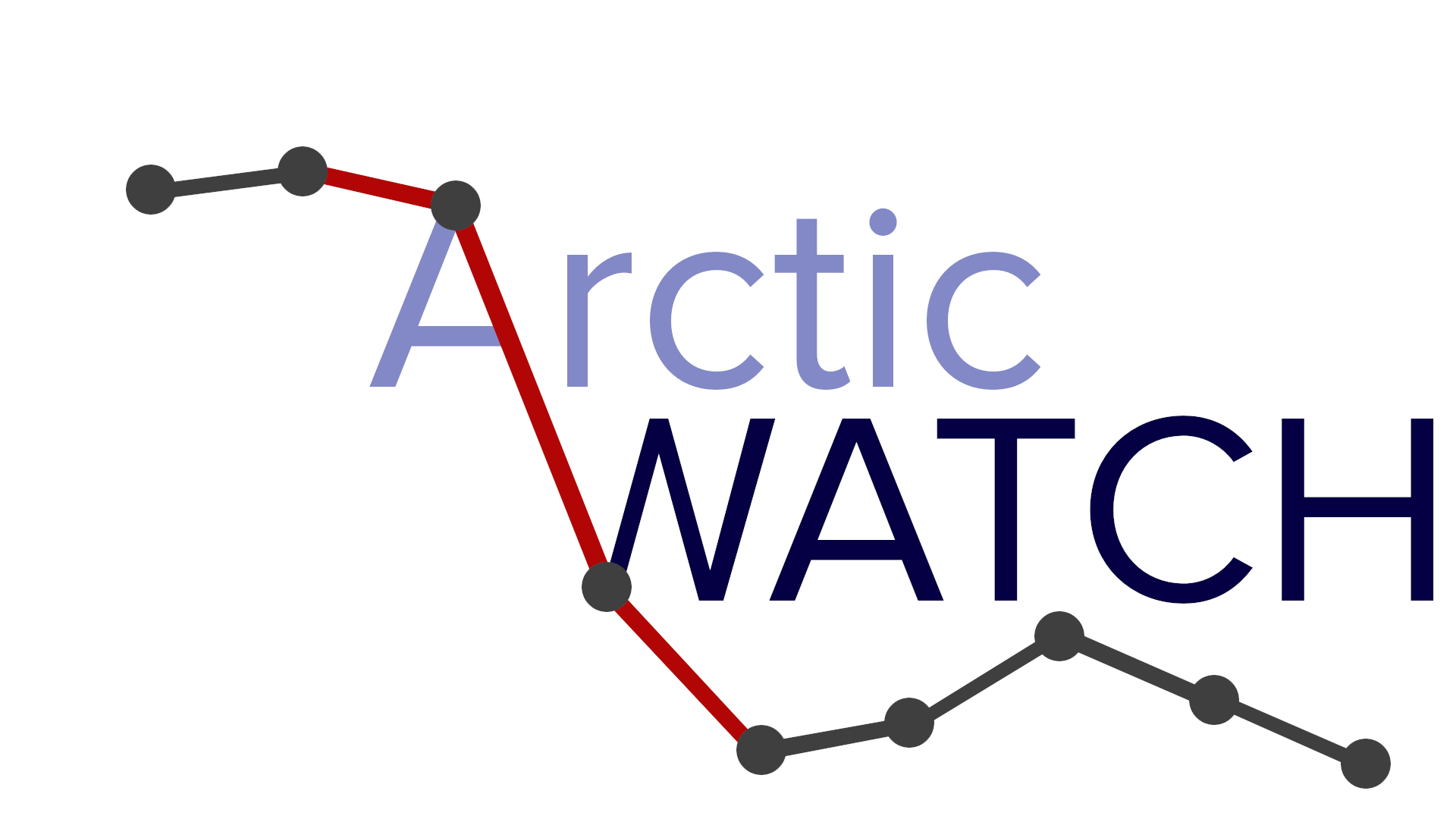
AI4InSync
Optimizing Southern Ocean observing systems using AI.
Contributors
François MassonnetTalks
KITP Polar 2025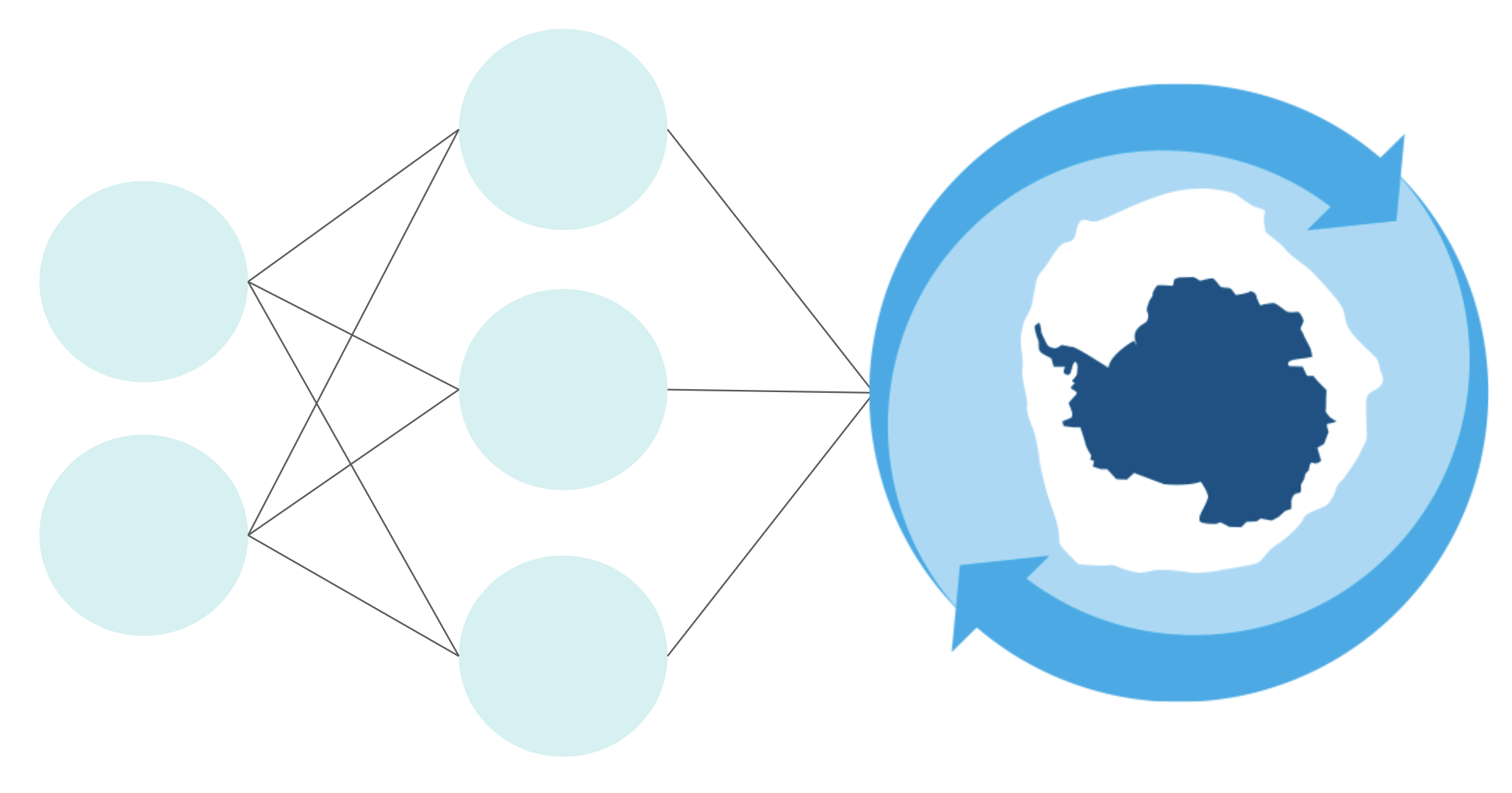
ArcticIceMotion
Machine learning forecasts of Arctic sea ice motion with XAI to understand drivers of variability
Contributors
Matthew Mazloff Sarah Gille Donata Giglio Patrick Heimbach Cecilia Bitz Kayli MatsuyoshiTalks
IARPC Modelers 2024 Liege Colloquium AI4ER Seminar 2023 ATOC Seminar 2023 Scientific ML Symposium 2023 ECCO 2022 OSM 2022 Polar AMS 2022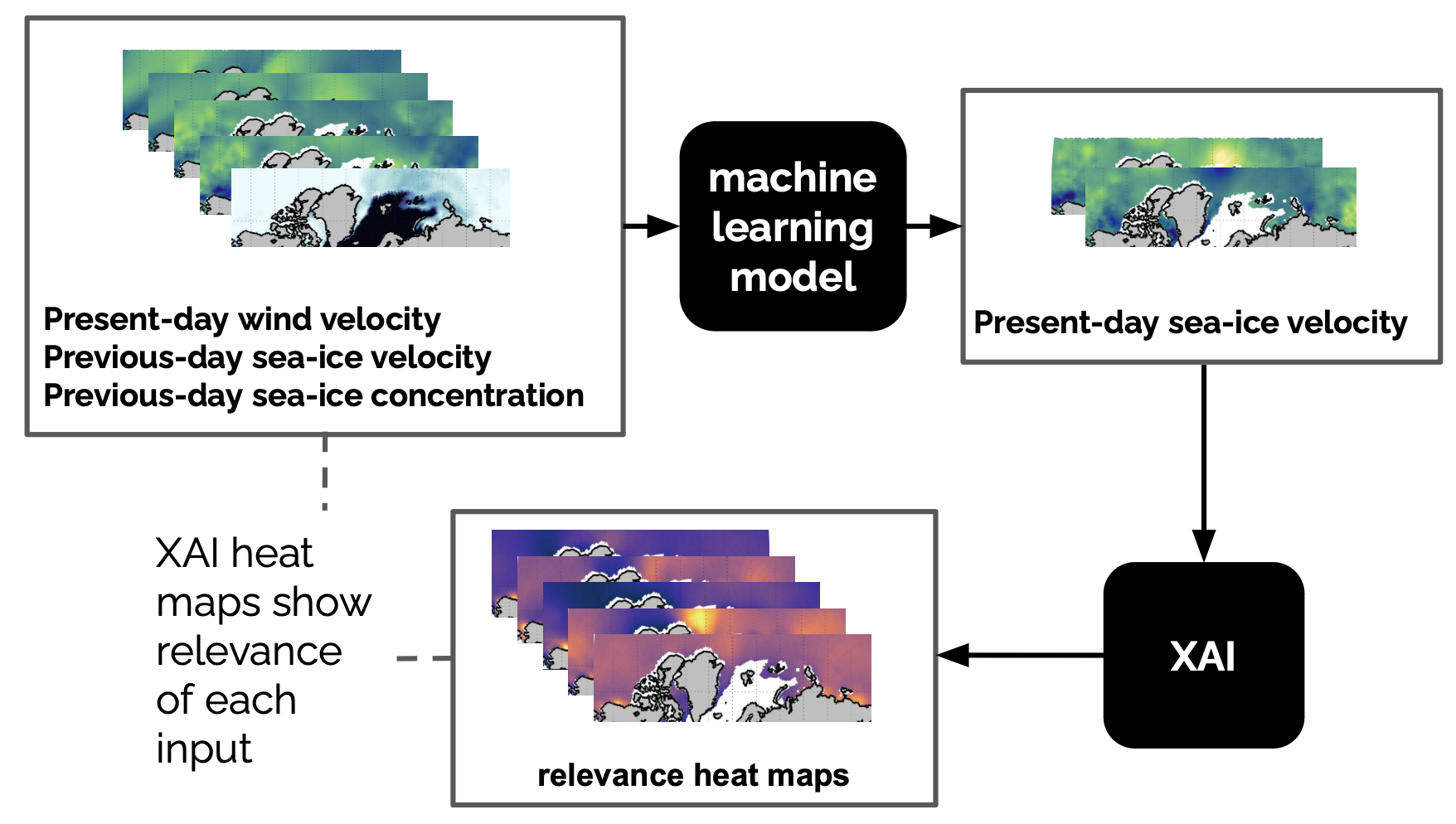
AROcean
Understanding the ocean salinity signature of atmospheric rivers in the California Current System
Contributors
Matthew Mazloff Sarah Gille Donata Giglio Aniruddh VaradarajanPublications
JPOTalks
FIRO 2022 CW3E Annual Meeting 2022 NOAA Science Seminar 2022 NASA Ocean Salinity 2022 AMS101 2021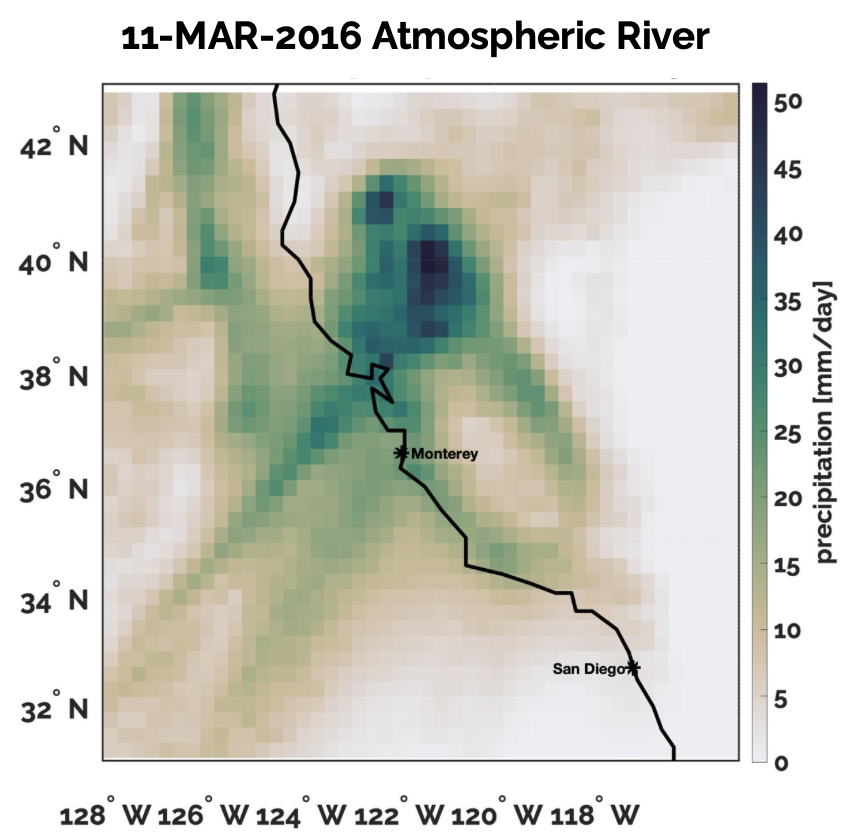